Meta
Instead of changing the data or learners in multiple ways and then see if fairness improves, postulate that the root causes of bias are the prior decisions that generated the training data. These affect (a) what data was selected, and (b) the labels assigned to the examples. They propose the \(\text{Fair-SMOTE}\) (Fair Synthetic Minority Over Sampling Technique) algorithm which (1) removes biased labels (via situation testing: if the model’s prediction for a data point changes once all of the data points' protected attributes are flipped, then that label is biased and the data point is discarded), and (2) rebalances internal distributions such that based on a protected attribute, examples are equal in both positive and negative classes. The method is just as effective in reducing bias as prior approaches, and its models achieve higher recall and F1 performance. Furthermore, \(\text{Fair-SMOTE}\) can simultaneously reduce bias for more than one protected attribute.
To overcome the “It is impossible to achieve fairness and high performance simultaneously (except in trivial cases)” truism, propose that extrapolating all variables by the same amount allows bias mitigation while maintaining/improving performance.
Sense-making
introduce Silva, an interactive system that helps users reason about unfairness in ML applications. Silva integrates a causality viewer to help identify the influence of potential bias, multi-group comparisons to help users compare subsets of data, and a visualization of metrics to quantify potential bias.
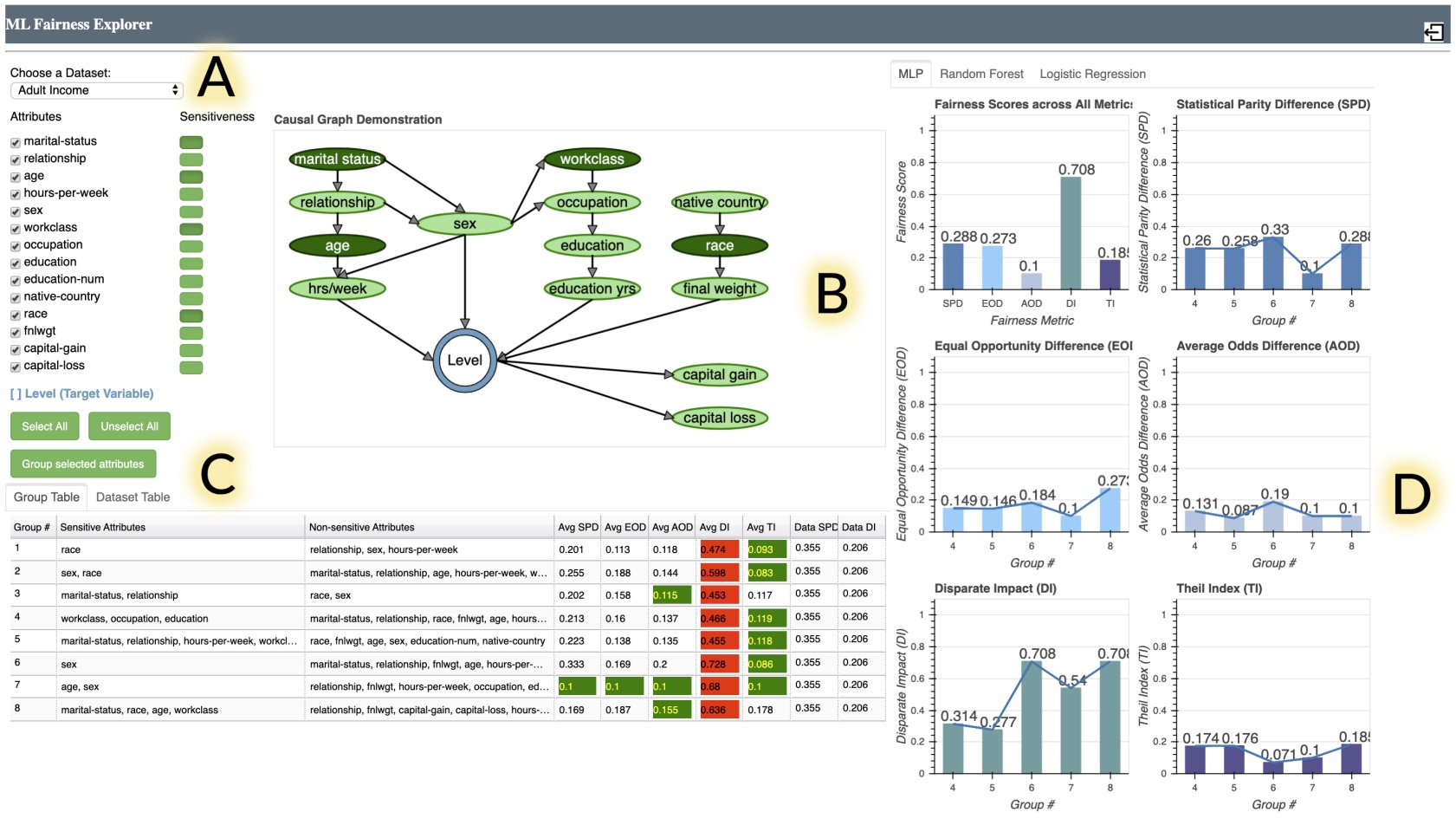
Time spent lurking HN makes me think that a theory for causality is yet to achieve consensus, e.g. The Book of Why: The New Science of Cause and Effect [pdf] | Hacker News .
are in the “causality expressed as directed acyclic graph (DAG)” camp. They define causal fairness as there being no path from sensitive / protected attributes to outcome variables in the DAG.
Through logs, think-aloud data and semi-structured interviews, explore how various interactive de-biasing tool affordances affect sense-making. Tools should test and account for the skill level of their users, e.g. users who didn’t understand Silva’s causal graph reported it not being useful, while users who were presented with recommendations may be subject to bias if untrained. Although combining exploration and recommendation risks choice overload, a hybrid approach may be useful, e.g. reducing switching costs, connecting individual UI components, and strategically offering recommendation when bootstrapping is involved. Exposing more model parameters, e.g. in Google’s What-If , distracted users from the de-biasing task.
Gender Bias
found two prominent facial analysis benchmarks being overwhelmingly composed of lighter-skinned faces. They then evaluated 3 commercial gender classification systems on a new facial analysis dataset that is balanced by gender and skin type. Darker-skinned females are the most misclassified (error-rates up to 34.7%), while the maximum error rate for lighter-skinned males was 0.8%.
Image captioning datasets, e.g. COCO, contain gender bias found in web corpora. They provide gender labels and split the COCO dataset to expose biased models. Models that rely on contextual cues (e.g. motorcycles tend to appear with men) fail more on the anti-stereotypical test data. They propose a model that provides self-guidance on visual attention to encourage the model to capture correct gender visual evidence.
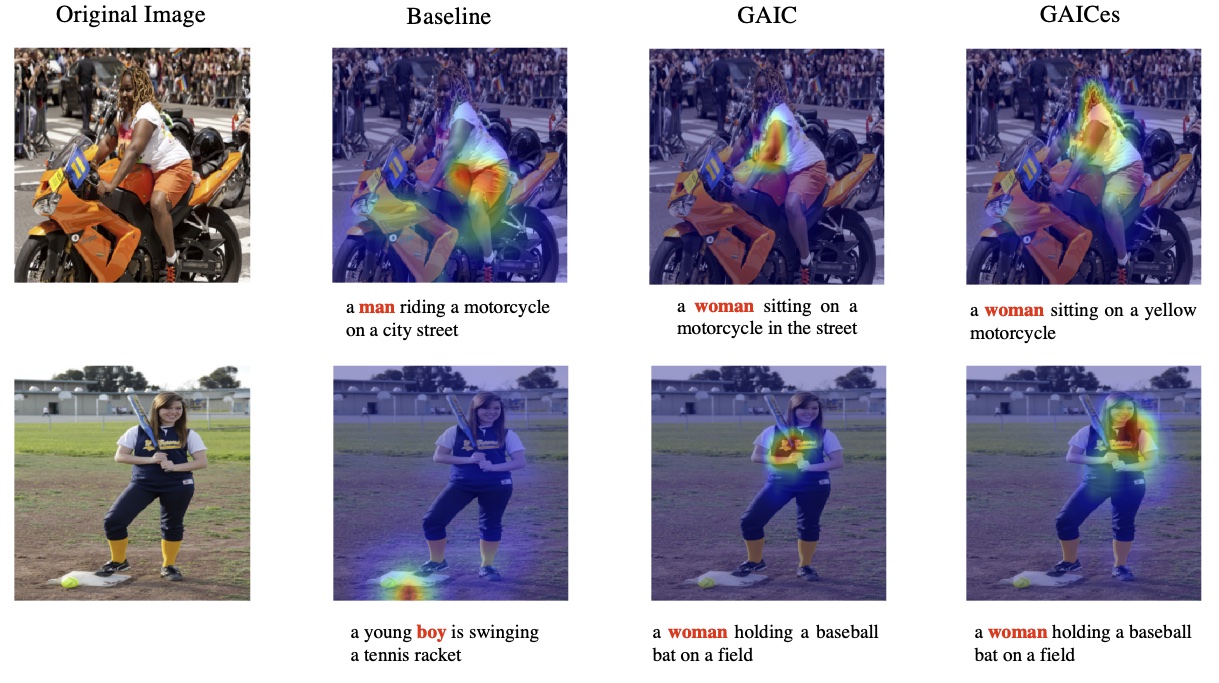
propose a framework that teaches the model to look at the correct part of the picture. That said, facial recognition software has its woes .
Recommendation Systems
#re-ranking #recommendation-system
show that active users, who are the minority, enjoy much higher recommendation quality than the majority inactive users. They propose a re-ranking approach by adding a fairness constraint. They group users into two groups in accordance with their activity levels, and aim to have the same recommendation quality for each group. This approach reduces the unfairness between advantaged and disadvantaged groups, and also improves overall recommendation quality, at the cost of sacrificing the recommendation performance of the advantaged group.
There’s a subtlety here. Although the active users are the minority, the recommender considers them the majority as they are the ones providing a lot of the training data.
Maybe recommendations based on collaborative filtering should also divide the user base into similar cohorts, and learning can take place within these cohorts? Hold up, doesn’t that happen by definition? If I watch movie A and someone else watched movies A and B, then the recommender can recommend B to me. argues that my perception is inaccurate. \(\Delta\)
Policy
Primary source of school district revenue in the US is public money. Existing school district boundaries promote financial segregation, with highly-funded school districts surrounded by lesser-funded districts and vice-versa. Authors propose the \({\rm F{\small AIR}\ P{\small ARTITIONING}}\) problem to divide a given set of nodes (e.g. schools) into \(k\) partitions (e.g. districts) such that the spatial inequality in a partition-level property (e.g. funding) is minimized. They show that \({\rm F{\small AIR}\ P{\small ARTITIONING}}\) is strongly NP-complete, and provide a reasonably effective greedy algorithm. They further provide an interactive website for exploring the impact of school redistricting.
Surprised that the authors of are affiliated with non-US institutions, but address a US problem. Fairness in the US school system is a gnarly problem, e.g. Boston Public Schools trying for equity with an algorithm .
Glossary
- Group Fairness
- The goal that based on the protected attribute, privileged and unprivileged groups will be treated similarly.
- Protected Attributes
- Features that may not be used as the basis for decisions, e.g. gender (and reassignment), race, religion, nationality, age, marital status, disability, socioeconomic status, pregnancy, sexual orientation. In an unfair ML system, the protected attribute divides the population into two groups (privileged and unprivileged) that have differences in terms of receiving benefits
References
- Fair Partitioning of Public Resources: Redrawing District Boundary to Minimize Spatial Inequality in School Funding. Mota, Nuno; Mohammadi, Negar; Dey, Palash; Gummadi, Krishna P.; Chakraborty, Abhijnan. The Web Conference, 2021. Max Planck Institute for Software Systems; Tehran Institute for Advanced Studies; Indian Institute of Technology. . doi.org . 2021. Cited 0 times as of Jan 23, 2022. scholar.google.com
- Gender shades: Intersectional accuracy disparities in commercial gender classification. Buolamwini, Joy; Timnit Gebru. Machine Learning Research: Fairness, Accountability and Transparency, Vol 1, pp. 77-91, 2018. Massachusetts Institute of Technology; Microsoft Research. . proceedings.mlr.press . Cited 2282 times as of Jan 23, 2022. scholar.google.com
- Bias in Machine Learning Software: Why? How? What to Do? Chakraborty, Joymallya; Suvodeep Majumder; Tim Menzies. European Software Engineering Conference and Symposium on the Foundations of Software Engineering, Proceedings of the 29th, August 2021, pp. 429–440. North Carolina State University. . doi.org . scholar.google.com . Cited 8 times as of Jan 23, 2022. github.com
also claim that they are one of the largest studies on bias mitigation yet presented. Data sets considered: UCI Adult , Compas , Statlog (German Credit Data) , Default of Credit Card Clients , Heart Disease , Bank Marketing UCI , Home Credit Default Risk , Student Performance , and Medical Expenditure Panel Survey .